Cancer is a highly complex disease, in part because of the ecosystems (or microenvironments) in which tumors reside. With a multitude of cell types and microorganisms, the tumor microenvironment can influence how cancer forms, whether it spreads, and how it responds to treatment. Making progress against cancer, therefore, will require a thorough understanding of the intricate relationships that exist between cancers and their microenvironments.
Fortunately, recent technological advances have enabled researchers to study the tumor microenvironment in unprecedented detail and decipher many of its complexities.
A plenary session at the American Association for Cancer Research (AACR) Annual Meeting 2024, titled “Profiling Tumor Ecosystems in Native Tissue Context,” spotlighted some of the innovative approaches used to examine the tumor microenvironment, as well as the discoveries these approaches have yielded thus far.
“Cancer is exceedingly complex, and it has taken the development of technologies that can understand cancer at this level of complexity to really start making in-roads,” said session moderator Christine Iacobuzio-Donahue, MD, PhD, a researcher at Memorial Sloan Kettering Cancer Center and the Editor-in-Chief of the AACR journal Cancer Research.
“I firmly believe we are well on our way to beating cancer because of … these types of approaches.”
Convergence of Multiple Technologies Provides Insight Into Distinct Cancers
Michael Angelo, MD, PhD, of Stanford University School of Medicine, shared a novel framework for studying spatial tumor biology, which he and colleagues used to understand disease progression and treatment in multiple cancer types.
Their multimodal framework relies on three methods to characterize components of the tumor microenvironment: multiplexed ion beam imaging by time of flight (MIBI-TOF) to map proteins, spatial transcriptomics to map gene expression, and matrix-assisted laser desorption/ionization (MALDI) to map glycan sugars in the tissue. They then employ machine learning to analyze the data at three spatial scales: the pixel level, the whole cell level, and the multicellular neighborhood level.
Angelo and colleagues applied their framework to characterize the expression patterns of seven common drug targets in glioblastoma. In doing so, the researchers uncovered opportunities to improve treatment efficacy for this highly fatal disease.
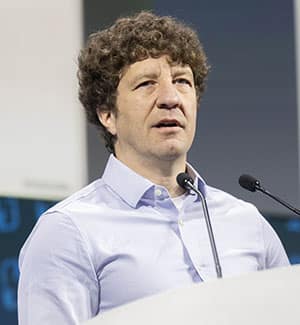
They found that when they treated tumors with two targeted therapy drugs instead of one, they were able to target about 20% more cancer cells. Increasing to three or more drugs, however, only marginally impacted the number of cells targeted. This was because a large portion of glioblastoma cells did not express any of the seven druggable targets.
Based on these findings, Angelo proposed that treatment for glioblastoma might benefit from a two-pronged strategy that combines dual-targeted therapy with interruption of immune suppression to enable clearance of cancer cells that lack druggable targets. To disrupt immune suppression, Angelo suggested targeting sialic acid-modified glycans, which he and colleagues found to be enriched in advanced glioblastomas.
Angelo and colleagues also applied their multimodal approach to identify spatial markers of disease progression in ductal carcinoma in situ (DCIS), a noninvasive form of breast cancer. By comparing tissue from patients whose DCIS did or did not progress to invasive breast cancer, the researchers identified multiple factors that increased the risk of progression.
These included changes to the spatial organization of 14 different cell populations, disruptions to the myoepithelium (a concentric tissue layer that separates DCIS cells from the surrounding stroma), and altered ductal topology. When they combined these determinants into a risk prediction profile, they were able to predict 10-year DCIS progression with an accuracy of 82%, Angelo reported.
Finally, he and colleagues analyzed metastatic triple-negative breast tumors to identify spatial features that could predict response to nivolumab and chemotherapy, which they used to develop a risk prediction model for disease relapse. The goal of such a model would be to identify patients who are most likely to benefit from the regimen before they begin treatment and avoid unnecessary treatment for those less likely to benefit.
However, the researchers found that, while their model accurately predicted relapse for patients already undergoing treatment, it had limited accuracy prior to treatment. These findings suggest that spatial determinants of response, such as those identified here, can change over the course of therapy, Angelo noted.
Regional Variation of Intratumoral Microbes Impacts Cancer Cell Functions
Microbes in the human body can have positive and negative impacts on human health. In the session’s second presentation, Susan Bullman, PhD, of the Fred Hutchinson Cancer Center, discussed how these microbes can impact cancer, sharing that the spatial distribution of microbes within tumors affects cellular functions.
She showed that Fusobacterium nucleatum were heterogeneously distributed across gastrointestinal tumors and that tumor regions enriched for the bacteria were immune suppressed and necrotic. Since necrosis results in reduced oxygen levels, necrotic regions are well suited for anaerobic bacteria such as F. nucleatum, Bullman noted.
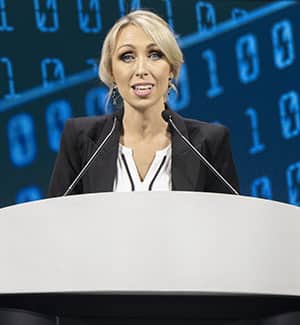
Using spatial transcriptomics and a newly developed method called INVADEseq, Bullman and colleagues found that the presence of bacteria altered gene expression of cells in the tumor microenvironment. As an example, F. nucleatum induced upregulation of cellular pathways associated with cell migration and metastasis in gastrointestinal cancers.
Bullman and colleagues also used microscopy techniques coupled with single-cell transcriptomics to simultaneously visualize bacterial populations and gene expression changes across colon cancers.
“Indeed, these intratumoral microbes are actively modulating their infected tumor niche within the tumor microenvironment,” she noted. She added that understanding how microbes impact cancer progression and treatment response may enable researchers to intercept unfavorable host-microbial interactions and improve patient outcomes.
Multimodal Spatial Transcriptomics to Understand Cancer Evolution
Joakim Lundeberg, PhD, of KTH Royal Institute of Technology and Science for Life Laboratory, discussed the power of combining spatial transcriptomics with other modalities to better understand cancer initiation and progression.
Early applications of spatial transcriptomics revealed the vast heterogeneity within cancer tissue, and combining conventional spatial transcriptomics with single-cell RNA sequencing provides even greater insight into the heterogeneous gene expression and cell types found in the tumor microenvironment, Lundeberg explained.
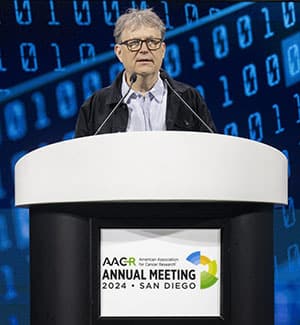
He and colleagues applied this approach to map the changes that occur as precancerous conditions evolve to prostate or breast cancer. They inferred gene copy number changes from gene expression data, with higher and lower gene expression potentially indicative of chromosome gains and losses, respectively.
They found that the frequency of inferred copy number changes increased as normal prostate tissue evolved to premalignant prostatic intraepithelial neoplasia (PIN) and then to prostate cancer. Further, they discovered that cells harboring these copy number changes were localized to glands.
In breast cancer tissue, Lundeberg and colleagues observed distinct inferred copy number changes when comparing noninvasive in situ breast cancer clones with invasive clones, with gains in chromosome 2 and chromosome 19, respectively.
Lundeberg also discussed the potential of spatial multiomics, which combines data on the spatial distribution of gene expression, metabolites, lipids, glycans, peptides, and histology, to identify biomarkers of cancer progression.
Lundeberg and colleagues are currently using deep learning to compile spatial multiomics data from tissue sections into three-dimensional tissue models to better understand the changes that occur across tumor tissues.
Adding a New Dimension to Studying Melanoma
While tissue profiling is conventionally performed on thin slices of tissue (typically 5 microns thick), Peter Sorger, PhD, of Harvard Medical School, argued that this approach is leading researchers to miss key information. Thin slices do not capture whole cells or even whole nuclei, he explained, so thicker sections are needed to properly characterize tissue pathology.
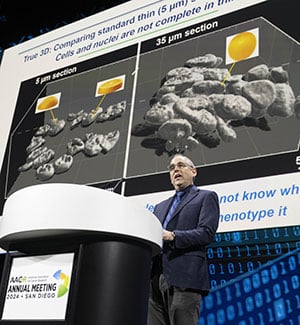
Sorger shared results from a newly developed method for the molecular profiling of tissue slices up to 35 microns in thickness. This allowed the researchers to examine cells across all three dimensions of melanoma tissues (length, width, and depth), which uncovered that the various cell populations that made up the tissue were densely packed and often intertwined—features that were not detected by conventional two-dimensional approaches.
An analysis of early melanoma tissue revealed heterogeneous expression of various immune markers, with inflammatory markers localized to sites where the melanoma had started to invade surrounding tissue. Further analysis showed that metastatic melanomas had similarly heterogeneous gene expression, which was organized around tissue vasculature, perinecrotic domains, and tumor margins.
Two-dimensional and three-dimensional imaging both have pros and cons, Sorger noted. Two-dimensional imaging has limited resolution but is simpler and can therefore be used to analyze a large number of specimens. In contrast, three-dimensional imaging provides deep, functional characterization but of a relatively small number of samples. Sorger hopes to find a way to combine the best of both techniques.
“We really now are pushing hard to … develop machine learning algorithms to pull these worlds together,” he said, noting that performing analyses with the depth of three-dimensional imaging but with the ease of two-dimensional imaging would greatly improve single-cell analyses.